Blogpost
Unlocking the Strategic Potentials of Big Data
Meet our authors
Reference items
Expert EN - Karsten Trostmann
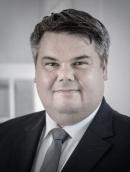
Expert Director
Karsten
Trostmann
Karsten Trostmann is an Expert Director at CORE. As a computer scientist, Karsten covers the main topics of IT strategy, evolutionary IT architecture, domain driven design, agile software developme...
Read moreKarsten Trostmann is an Expert Director at CORE. As a computer scientist, Karsten covers the main topics of IT strategy, evolutionary IT architecture, domain driven design, agile software development, and cloud infrastructures. Karsten’s previous project experience at CORE includes evaluating the platform strategy for a digital insurer, developing cloud operations for an identity provider, and architecture reviews in various projects.
Read less