Blogpost
From theory to practice. Current artificial intelligence models in the finance industry
Meet our authors
Reference items
Expert EN - Julius Heitmann
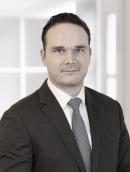
Expert Director
Julius
Heitmann
Julius Heitmann is an Expert Director at CORE. He has realised various AI and analytics projects in the banking, insurance and medical sectors. Julius not only advises our clients but also develops...
Read moreJulius Heitmann is an Expert Director at CORE. He has realised various AI and analytics projects in the banking, insurance and medical sectors. Julius not only advises our clients but also develops hands-on software for CORE that makes our everyday consulting work more efficient. Based on his diverse experience, Julius can point out strategic perspectives as well as accompany concrete implementations.
Read less